BENEFRI Summer School 2024
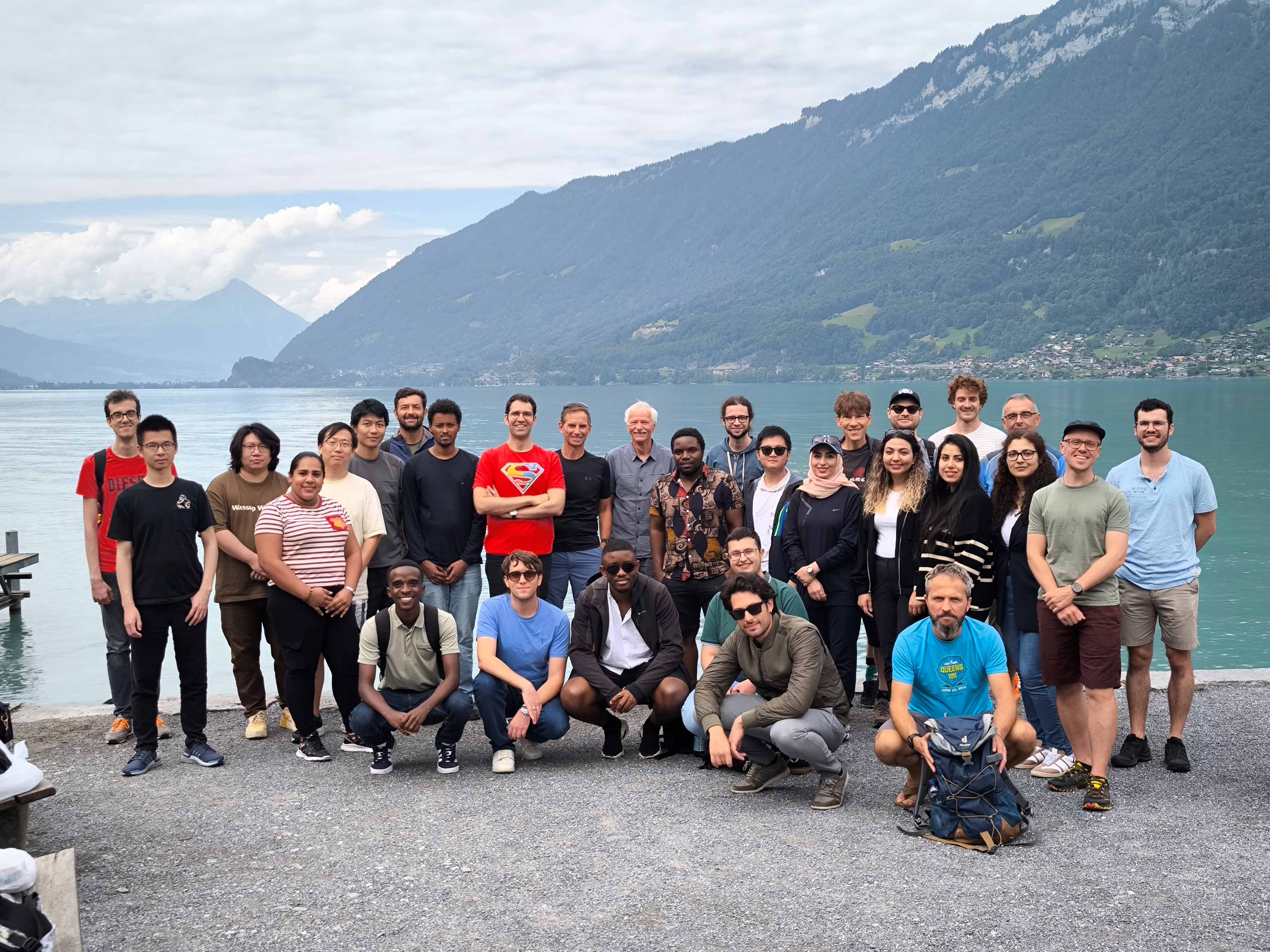
Organization
The workshop is organized by the University of Bern (Prof. Dr. Torsten Braun, Dr. Antonio Di Maio)
When
The summer school will take place in the city of Brienz from Monday, 2 September 2024 to Wednesday, 4 September 2024.
Where
Lindenhof Hotel, Lindenhofweg 15, CH-3855 Brienz
Link to Hotel website: https://hotel-lindenhof.ch/
How to get there?
With public transportation from Bern: 9.04 train IC81 to Interlaken Ost, arriving at 9.58 to Interlaken Ost. Then, change on train PE direction Luzern from Interlaken Ost at 10.04 to Brienz, arriving at 10.21. Then 10-minute walk (449 meters) to the Hotel address.
Participants List (28 people)
Students Bern (13): Elham Hashemi Nezhad, Ivonne Nunez, Zimu Xu, Hexu Xing, Chuyang Gao, Mingjing Sun, Solomon Fikadie Wassie, Jinxuan Chen, Sajedeh Norouzi, Jesutofunmi Ajayi, Yamshid Farhat, Fabrice Marggi, Zahra Khodadadi
Students Fribourg (2): Yann Maret, Frédéric Montet
Students Neuchâtel (9): Romain Claret, Peterson Yuhala, Simon Queyrut, Pasquale De Rosa, Mpoki Mwaisela, Louis Vialar, Romain de Laage, Abele Malan, Andrea de Murtas
Senior Bern (3): Torsten Braun, Antonio Di Maio, Eric Samikwa
Senior Fribourg (2): Sébastien Rumley, Jean Hennebert
Senior Neuchâtel (3): Pascal Felber, Peter Kropf, Valerio Schiavoni
Notes for speakers
Each talk will be 15 minutes long, followed by a 10-minute Q&A session.
Please spontaneously organize to share the same laptop among all presenters of a session to minimize the context switch penalty.
Program
Monday, 2 September 2024
Time | Content |
---|---|
13.45 - 14.00 | --- Welcome --- |
14.00 - 15.30 | Session 1: Distributed Artificial Intelligence (chair: Antonio Di Maio) |
14.00 | Mingjing Sun (UniBE) - Personalized Decentralized Learning for Mobile Virtual Reality Networks |
14.30 | Simon Queyrut (UniNE) - Federated Theft of cGANs |
15.00 | Ivonne Nunez (UniBE) - Privacy Protection and Efficient Energy Metering through Federated Learning in Smart Homes |
15.30 | Zimu Xu (UniBE) - Communication-Efficient Federated Learning for Scalable Psychological Monitoring in High-Density Areas |
16.00 | --- Coffee break (included) --- |
16.30 - 19.00 | Session 2: Networks and AI (chair: Eric Samikwa) |
16.30 | Solomon Fikadie Wassie (UniBE) - Dynamic VNF deployment and SFC reconfiguration in 6G Network Architecture |
17.00 | Elham Hashemi Nezhad (UniBE) - Decentralized Orchestration of RAN Controllers in 6G Networks |
17.30 | Romain Claret (UniNE) - From Neocortical Columns to Humanity-Inspired AI Systems |
18.00 | Frédéric Montet (HEIA-FR) - Enabling Diffusion Model for Conditioned Time Series Generation |
18.30 | Dr. Yann Maret (HEIA-FR) - Improving the Performance of MANETs using Machine Learning |
19.00 - 20.00 | Social Time |
20.00 | --- Dinner (included, except alcoholic beverages, in the hotel's restaurant) --- |
Tuesday, 3 September 2024
Time | Content |
---|---|
7.30-8.30 | --- Breakfast (included) --- |
8.30-10.30 | Session 3: Intelligent Network Management I (chair: Pascal Felber) |
8.30 | Chuyang Gao (UniBE) - Dual-Engine Intelligent Caching: A Joint Optimization Framework for 360-degree Mobile VR Video Edge Caching |
9.00 | Hexu Xing (UniBE) - Multi-Agent Reinforcement Learning for Enhanced Network QoS Optimization |
9.30 | Romain de Laage (UniNE) - Privacy-preserving map-reduce protocol with distrustful parties |
10.00 | --- Coffee break (included) --- |
10.30-12.00 | Session 4: Intelligent Network Management II (chair: Valerio Schiavoni) |
10.30 | Sajehed Norouzi (UniBE) - From CNN to GNN: Advancing Channel Estimation in Massive MIMO Communication Systems |
11.00 | Jinxuan Chen (UniBE) - Federated Split Learning for Multi-Modal Beamforming |
11.30 | Abele Malan (UniNE) - More efficient and expressive graph generation with hybrid diffusion |
12.00-19.00 | --- Lunch (not included) and social activity: Boat trip (ticket not included) leaving 12.40 from Brienz to Iselwald and Hike from Iselwald to Giessbach (around 2h30m) |
19.00 | --- Dinner (included, except alcoholic beverages, restaurant Weisses Kreuz, Brienz) --- |
Wednesday, 4 September 2024
Time | Content |
---|---|
7.30-8.30 | --- Breakfast (included) --- |
8.30 - 10.30 | Session 5: Trust and Systems I (chair: Jean Hennebert) |
8.30 | Andrea De Murtas (UniNE) - ConFaaS: easy execution and evaluation of cloud-native workloads on Confidential VMs |
9.00 | Pasquale De Rosa (UniNE) - On the Cost of Model-Serving Frameworks |
9.30 | Zahra Khodadadi (UniBE) - Improving IoRT Networks: Cross-Tier Resource Allocation for Multi-Antenna UAV Relays in Space-Air-Ground Integrated Networks |
10.00 | Tofunmi Ajayi (UniBE) - Hierarchical Learning for Network Slice Provisioning |
10.30 | --- Coffee break (included) --- |
11.00-13.00 | Session 6: Trust and Systems II (chair: Sebastien Rumley) |
11.00 | Louis Vialar (UniNE) - BlindexTEE: Leveraging Trusted Execution Environments to enable End-To-End Database Encryption |
11.30 | Yamshid Farhat (UniBE) - Synthetic Consumption Profile Generation using Generative AI for Electric Network Planning |
12.00 | Fabrice Marggi (UniBE) - TBD |
12.30 | Mwaisela Mpoki (UniNE) - Evaluating the Potential of In-Memory Processing to Accelerate Homomorphic Encryption |
--- Farewell and final remarks --- |
Presentations Abstracts
University | Name | Surname | Title | Abstract |
UniBE | Tofunmi | Ajayi | Hierarchical Learning for Network Slice Provisioning |
In this presentation, we address the challenge of provisioning network slices in edge-enabled networks as part of the slice orchestration process. Specifically, we focus on the placement of diverse service function chains in such networks. We propose a Hierarchical Bandit Learning solution that sequentially learns an SFC placement policy by addressing a combinatorial optimization problem using multiple agents in the network. Our results show that our approach is able to achieve good results in accepting slice requests and efficiently utilizing network resources. |
UniBE | Jinxuan | Chen | Federated Split Learning for Multi-Modal Beamforming |
The use of multi-modal sensors has the potential to improve the efficiency of beamforming due to the availability of the situational information acquired by monitoring sensors. However, the raw data collected by multimodal sensors may be in large quantities or sensitive to transmit over the network to a central server. On the other hand, large state-of-the-art DNN models are typically required to process the sensor data, leading to high computational demands on the user devices. To achieve efficient distributed training using multi-modal sensor data, we propose the use of Federated Split Learning for multi-modal beamforming. The evaluation results are based on the real-world datasets which proves the robustness and the efficiency of our proposed methods. |
UniNE | Romain | Claret | From Neocortical Columns to Humanity-Inspired AI Systems |
This presentation unveils an ongoing research project aimed at developing an open-ended neuroevolution framework that pushes the boundaries of AutoML, drawing inspiration from neocortical columns and humanity-inspired AI systems. The proposed paradigm seeks to eliminate the need for human engineering in model development by creating a system that autonomously learns and evolves based solely on input data, ground truth, and self-generated synthetic data. The framework comprises three main components: evolving auto-tuned neural networks, evolving columnar neural networks, and evolving collaborating neural networks. Initial results on the first component demonstrate the effectiveness of ES-HyperNEAT hyperparameter optimization using a Tree-structured Parzen Estimator (TPE) on MNIST and the transferability of these hyperparameters to other tasks. Ongoing work expands this approach with additional hyperparameters, tasks, and search methods to gain insights and extend our dataset to develop models capable of offline and online self-tuning. Inspired by neocortical columns, ongoing work on the second component involves evolving self-distributed models for feature extraction and learning as column networks. These low-level feature extraction models self-recombine to achieve higher levels of complexity in feature extraction, similar to Convolutional Neural Networks but with the added capability of parallel processing, mimicking the distributed nature of cortical information processing. The third component will enable communication and specialization among columnar networks, allowing them to tackle larger tasks and share or fine-tune low-level models akin to the collaborative nature of cortical regions in the human brain. By integrating these three components, the framework aims to create an autonomous and adaptive machine-learning system that aims to mirror human cognition's efficiency and adaptability. The ultimate goal: evolve neural networks, create iteratively self-improving AI, emulate human-like learning capabilities, ..., and profit. |
UniNE | Romain | de Laage | Privacy-preserving map-reduce protocol with distrustful parties |
A requestor wishes to ask the parties for specific information (for example, the average salary in a professional sector), the parties want to participate to the computation but don't want to reveal the information they hold with others. We want to explore the benefits of trusted execution environments (TEE) for computing data securely with distrustful parties. We define an architecture and different variants to measure the impact of different techniques in terms of performance and security. The work will compare combination of techniques based on threshold fully homomorphic encryption and TEEs with different security model. |
UniNE | Andrea | De Murtas | ConFaaS: easy execution and evaluation of cloud-native workloads on Confidential VMs |
In cloud computing, ensuring the security and confidentiality of workloads is essential. Trusted Execution Environments (TEEs) have been emerging as powerful tools to achieve this by providing secure and isolated environments for workload execution. Major cloud providers are increasingly offering TEE-powered machines that leverage next-generation TEEs, such as Intel Trust Domain Extensions (TDX) and AMD Secure Encrypted Virtualization with Secure Nested Paging (SEV-SNP). These advancements focus on the creation and management of confidential virtual machines, rather than secure regions for executing parts of applications as seen with previous technologies like Intel SGX and Arm TrustZone. Additionally, TEEs have potential applications in serverless computing, where infrastructure is fully managed by the cloud provider, who could allow users to run workloads in a TEE. This paper presents a prototype framework as a proof of concept for handling and dispatching confidential workloads in a Function-as-a-Service (FaaS) setting. This framework is focused on easily running user-defined functions while performing an evaluation of the performance of the various platforms. Prior to the FaaS evaluation, preliminary measurements for a variety of tasks-such as machine learning inference, SQLite benchmarking, OS operations benchmarking, and attestation were performed. Our evaluation covers Intel's TDX, AMD's SEV-SNP, and ARM's Confidential Compute Architecture (CCA), the latter assessed through simulation due to the lack of available hardware. |
UniNE | Pasquale | De Rosa | On the Cost of Model-Serving Frameworks |
In machine learning (ML), the inference phase is the process of applying pre-trained models to new, unseen data with the objective of making predictions. During the inference phase, end-users interact with ML services to gain insights, recommendations, or actions based on the input data. For this reason, serving strategies are nowadays crucial for deploying and managing models in production environments effectively. In this work, I evaluate the performances of five widely-used model serving frameworks (TensorFlow Serving, TorchServe, MLServer, MLflow, and BentoML) under four different scenarios (malware detection, cryptocoin prices forecasting, image classification, and sentiment analysis). |
UniBE | Yamshid | Farhat | Synthetic Consumption Profile Generation using Generative AI for Electric Network Planning |
The generation of synthetic profiles is crucial for electric network planning, as it helps identify necessary infrastructure expansions to ensure a high quality of supply for all customers. Traditional methods focusing on clustering are suboptimal because average profiles tend to smooth out local power peaks and fail to accurately represent simultaneity factors among customers at different levels of aggregation—key elements for optimal network planning. In contrast, generative methods, particularly diffusion models, have emerged as promising approaches for creating synthetic time-series profiles of electricity consumption behavior. This review will explore the potential of these generative methods, highlighting their advantages over conventional approaches. Additionally, it will assess existing evidence of their effectiveness using a proposed review framework designed to evaluate the quality of synthetic profile generation. |
UniBE | Chuyang | Gao | Dual-Engine Intelligent Caching: A Joint Optimization Framework for 360-degree Mobile VR Video Edge Caching |
360-degree video edge caching has become an effective solution for minimizing delay to popular content. We propose a dual-engine intelligent caching framework that synergizes operations research and deep reinforcement learning for 360-degree video edge caching and content prefetching. This framework introduces a shared hierarchical caching architecture and formulates a global shared caching placement problem. Adopting a cutting-edge pruning approach, the local cache replacement algorithm is proposed. This utilizes deep reinforcement learning to predict cache boundaries as cutting planes, thereby efficiently pruning the search space of Integer Linear Programming. Numerical results show that proposed scheme provides significantly improved performance relative to alternative caching schemes. |
UniBE | Elham | HashemiNezhad | DERRIC: Decentralized Reinforced RAN Intelligent Controller Orchestration for 6G Networks |
We present a decentralized orchestration and distributed controllers method using Reinforcement Learning (RL) to make flow processing intelligent, reliable, and fast. In order to illustrate the role of intelligence in the method, we propose a multi-agent approach to observe the state in each time step to deploy controllers in the network. After controller placement, again, we utilize RL to assign Transmission Power to each user by the controllers. |
UniBE | Zahra | Khodadadi | Improving IoRT Networks: Cross-Tier Resource Allocation for Multi-Antenna UAV Relays in Space-Air-Ground Integrated Networks | In response to reducing power consumption while reducing equipment and maintaining high data rate requirements in space-air-ground integrated networks (SAGIN) as part of IoRT networks, I present a system model and optimization scheme. This approach involves multi-antenna UAV relays, selected gateways, and a combination of RF and FSO links. The optimization problem aims to minimize the weighted sum of the number of gateways and the total UAV power consumption by jointly optimizing UAV deployment, UAV power allocation, gateway selection, and channel allocation. Through the joint adoption of size-constrained PSO-K-means clustering, simulated annealing (SA) method, and successive convex approximation (SCA) method, we address the NP-hard and non-convex problem effectively. I present the results of our simulations, demonstrating the effectiveness of our proposed scheme in achieving optimal clustering, UAV deployment, and gateway selection. The study determines the required number of gateways for different scenarios. For instance, almost all UAVs are selected as gateways to minimize UAV power consumption without considering any constraint on the number of gateways. Additionally, the performance difference between considering total UAV power consumption and only transmission power in the system is highlighted. The results also underscore the impact of the number of UAVs and the number of their antennas on system performance. |
UniNE | Abele | Malan | More efficient and expressive graph generation with hybrid diffusion |
Existing attributed graph generative models rapidly become costly for non-geometric graphs with hundreds of nodes and cannot generate complex node information. To help alleviate these issues, we propose a new model architecture for hybrid denoising diffusion on a compressed graph structure with latently represented node features. We combine discrete and continuous diffusion to help maintain the structural sparsity of edges and allow encoding high dimensional multivariate features within nodes, respectively. Furthermore, we use a structurally lossless scheme to compress adjacent node pairs, reducing up to half of the nodes from the initial graph. We also employ a graph variational autoencoder to compactly represent concatenated categorical and continuous features. Current results on data from domains like social networks show that the approach can allow synthesizing graphs with high-fidelity node data while maintaining similar connectivity statistics to prior models and reducing computation. |
HEIA-FR | Yann | Maret | Improving the Performance of MANETs using Machine Learning |
This work is concerned with the improvement of the overall performance and Quality of Service (QoS) in Mobile Ad-hoc Networks (MANETs). It proposes a 3-cross-layer optimization scheme which maximizes the Completion Ratio (CR) and minimizes the Round-Trip-Time (RTT). It is focusing on optimizing the routing, scheduling and the flow control using machine learning. Specific requirements have been articulated in a number of scenarios and these have been employed to measure the performance of the proposed 3-cross-layer optimization scheme. The open Anglova.net is a scenario which matches the requirement of the research study funded by Armasuisse. This scenario has been used to test advanced cross layer algorithms. The 6-laptop is another scenario which has been used to measure the performance of routing protocols. There was an attempt to contrast these two scenarios, however, and despite that the performance comparison has been made these are considered valid in their own merit. The theoretical performance has been evaluated with fitted radio models. The scope was to provide emulation results from realistic radio models. Omniscient approaches such as the Omniscient Dijkstra Routing balanced (ODRb) and an omniscient suboptimal Time Division Multiple Access (TDMA) schedule are assessed on the dynamic Anglova.Net scenario to evaluate close to optimal performance in real time emulation using the Extendable Mobile Ad-hoc Network Emulator (EMANE). The omniscient agent called Graph Neural Network (GNN-t) is proposed to seek alternative longer routes and reduced congestion. For realistic distributed MANETs, Optimized Link State Routing (OLSR) is investigated and enhanced for multi-hop networks with 24 nodes or less. The distributed routing OLSRv2 is improved using (1) Signal to Interference plus Noise Ratio (SINR) to estimate the link quality, (2) stability of the Multipoint Relays (MPRs) to improve route dissemination and (3) advanced link cost computations to offer reliable routes. The distributed node view graph provided by the routing protocol is exploited to compute schedules to seek theoretical performance. Four scheduling schemes are proposed based on the node view graph: (1) an oblivious to traffic schedule, (2) an advertised traffic based schedule, (3) a slot request algorithm with retransmissions and (4) a ML based 2-hop scheduling scheme. A Flow Control (FC) scheme at the network layer was proposed to reduce the user traffic when node congestion occurs. It maintains the packets on the node during disconnections. OLSR+SINRT, a deterministic and enhanced version of OLSRv2d using SINR information, increases CR from 66% to 76% on the Anglova scenario with fading. Measurements were conducted to assess the performance of OLSR+SINRT (CR=81%) and OLSR (CR=79%) in indoor environments. Results demonstrate major improvements using routing and scheduling with omniscient and distributed solutions in realistic scenarios. |
UniBE | Fabrice | Marggi |
Leveraging Secondary Data and ML Techniques to Predict Mobility Patterns in Sparse Data Regions |
The increasing variability of demand facing transport systems is giving rise to the necessity for changes to infrastructure to address the shifting needs of users. However, in rural and regional areas, databases that could explain and predict mobility patterns are often limited and sparse. The present work proposes an approach combining data fusion techniques, namely transfer learning to enhance individual data sources and federated learning to integrate the different information. The objective of this approach is to integrate traffic measurements with secondary data sources that indirectly reflect mobility trends to refine insights into mobility patterns. The objective is to construct a comprehensive digital twin of the transport system and its surrounding environment within a use case of a touristic region. This will result in the creation of a robust tool for the effective management and anticipation of transport demand and the need for infrastructure. |
HEIA-FR | Frédéric | Montet | Enabling Diffusion Model for Conditioned Time Series Generation |
Synthetic time series generation is an emerging field of study in the broad spectrum of data science, addressing critical needs in diverse fields such as finance, meteorology, and healthcare. In recent years, diffusion methods have shown impressive results for image synthesis thanks to models such as Stable Diffusion and DALL·E, defining the new state-of-the-art methods. In time series generation, their potential exists but remains largely unexplored. In this work, we demonstrate the applicability and suitability of diffusion methods for time series generation on several datasets with a rigorous evaluation procedure. Our proposal, inspired from an existing diffusion model, obtained a better performance than a reference model based on generative adversarial networks (GANs). We also propose a modification of the model to allow for guiding the generation with respect to conditioning variables. This conditioned generation is successfully demonstrated on meteorological data. |
UniNE | Mwaisela | Mpoki | Evaluating the Potential of In-Memory Processing to Accelerate Homomorphic Encryption |
The widespread adoption of cloud-based solutions introduces privacy and security concerns. Techniques such as homomorphic encryption (HE) mitigates this problem by allowing computation over encrypted data without the need for decryption. However, the high computational and memory overhead associated with the underlying cryptographic operations has hindered the practicality of HE-based solutions. While a significant amount of research has focused on reducing computational overhead by utilizing hardware accelerators like GPUs and FPGAs, there has been relatively little emphasis on addressing HE memory overhead. Processing in-memory (PIM) presents a promising solution to this problem by bringing computation closer to data, thereby reducing the overhead resulting from processor-memory data movements. In this work, we evaluate the potential of a PIM architecture from UPMEM for accelerating HE operations. Firstly, we focus on PIM-based acceleration for polynomials operations, which underpin HE algorithms. Subsequently, we conduct a case study analysis by integrating PIM into two popular and open-source HE libraries, OpenFHE and HElib. Our study concludes with key findings and takeaways gained from the practical application of HE operations using PIM, providing valuable insights for those interested in adopting this technology. |
UniBE | Sajedeh | Norouzi | From CNN to GNN: Advancing Channel Estimation in Massive MIMO Communication Systems |
Recent advancements in deep neural networks (DNNs) have significantly enhanced channel estimation (CE) for massive multiple-input multiple-output (MIMO) communication systems. Despite these improvements, challenges such as complexity reduction and robustness enhancement remain. Additionally, existing methods often lack clarity on which channel features are critical for DNN-based denoising. This paper addresses these issues by first analyzing the strengths and limitations of current deep learning-based CE techniques, including Convolutional Neural Networks (CNNs) like SRCNN and DNCNN, across various domains. We then introduce a novel graph neural network (GNN) approach for CE that integrates the graph topology of the system into the neural network architecture. This GNN model learns the mapping from the initial estimated channel matrix to the true channel matrix, leveraging permutation equivariance and robust generalization properties. Simulation results demonstrate that the proposed GNN-based method effectively generalizes across varying numbers of antennas and consistently delivers high performance. |
UniBE | Ivonne | Nunez | Privacy Protection and Efficient Energy Metering through Federated Learning in Smart Homes |
This study explores using FL in smart energy metering in connected homes, focusing on user privacy protection. It analyzes how FL and clustering techniques can improve the accuracy of energy consumption prediction models, considering variables such as the presence of solar panels, household appliances, electric vehicles, and the number of inhabitants. Furthermore, possible privacy attack mechanisms in this context are investigated, such as identifying individual devices from aggregated data from a single smart meter. This highlights the need for privacy-safeguarding solutions in advanced metering scenarios. |
UniNE | Simon | Queyrut | Federated Theft of cGANs |
Conditional Generative Adversarial Networks (cGANs) are increasingly popular web-based synthesis services accessed through a query API, e.g. cGANs generate a cat image based on a "cat" query. However, cGAN-based synthesizers can be stolen via adversaries' queries, i.e. model thieves. The prevailing adversarial assumption is that thieves act independently: they query the deployed cGAN (i.e. the victim), and train a stolen cGAN using the images obtained from the victim. A popular anti-theft defense consists in throttling down the number of queries from any given user. We consider a more realistic adversarial scenario: model thieves collude to query the victim, and then train the stolen cGAN. ClueS is a new federated model stealing framework, enabling thieves to bypass throttle-based defences and steal cGANs more efficiently than through individual efforts. |
UniBE | Mingjing | Sun | Personalized Decentralized Learning for Mobile Virtual Reality Networks |
In the era of immersive technologies, Mobile Virtual Reality (VR) networks are gaining significant traction, demanding efficient and adaptive learning mechanisms to handle diverse user interactions and dynamic network conditions. This paper introduces a novel approach called Personalized Decentralized Learning (PDL) for Mobile VR networks, aimed at enhancing the performance and user experience of VR applications. PDL leverages decentralized learning paradigms to enable individual VR devices to collaboratively and independently learn from their interactions and environment without relying on a central authority. By integrating personalization techniques, each device tailors its learning process to accommodate user preferences and contextual factors, resulting in improved content delivery, reduced latency, and enhanced user satisfaction. The proposed method is evaluated through real-world VR dataset OpenEDS, demonstrating its effectiveness in optimizing network resources and adapting to varying VR scenarios. The results highlight the potential of PDL to transform Mobile VR networks into more resilient and user-centric systems, paving the way for advanced immersive experiences. |
UniNE | Louis | Vialar | BlindexTEE: Leveraging Trusted Execution Environments to enable End-To-End Database Encryption |
Using cloud-based applications comes with privacy implications, as the end-user looses control over their data. |
UniBE | Solomon | Wassie | Dynamic VNF deployment and SFC reconfiguration in 6G Network Architecture |
The deployment locations of Virtual Network Functions (VNFs) in distributed edge and cloud data centers significantly impact network performance and Quality of Service metrics, such as delay and throughput, thereby affecting overall user service requests. Traditionally, network functions like Network Address Translations (NATs) and firewalls were supported by dedicated and static hardware. However, there is now a substantial shift towards deploying VNFs on dynamically reconfigurable, cost-effective general-purpose servers, enabling greater adaptability and efficiency in managing network resources. Cloud computing facilitates the borderless and dynamic deployment and migration of VNFs, optimizing network performance. User mobility, hardware failure, traffic performance requirements, and load balancing can trigger network service migration. Machine Learning techniques have emerged as promising tools for decision-making, enabling seamless automation of network services by learning optimal decision policies from data. To achieve scalability and flexibility for both network operators and user traffic, VNFs are deployed in the form of Service Function Chains (SFCs), allowing dynamic service relocation, enhancing performance, reducing latency, and lowering costs. Efficient service deployment is essential for optimal operations and quality of service optimization. To meet quality of service requirements, VNFs must be optimally chained and placed.Deep reinforcement learning (DRL) algorithms, using deep neural networks (DNNs), develop optimal control policies from historical data and perform well in complex environments. This study leverages DRL to enhance VNF placement and SFC management, improving network performance and reducing costs. |
UniBE | Hexu | Multi-Agent Reinforcement Learning for Enhanced Network QoS Optimization |
Ensuring the Quality of Service (QoS) for diverse services within a network presents a significant challenge, particularly in modern architectures like Software-Defined Networking (SDN), where manual configuration is often required. This process is not only slow and prone to errors but also struggles to adapt to dynamic network conditions. In this paper, we propose a multi-agent reinforcement learning (MARL) approach to automate and optimize QoS management across network nodes. By modeling each network node as an independent agent, we train these agents to collaborate and compete, thereby achieving an optimal balance of resource allocation that meets the overall QoS requirements. Our approach not only enhances resource allocation efficiency but also significantly reduces response times compared to traditional methods. Experimental results demonstrate the effectiveness and adaptability of our solution. |
|
UniBE | Zimu | Xu |
Communication-Efficient Federated Learning for Scalable Psychological Monitoring in High-Density Areas |
Federated learning is widely applied in privacy-sensitive domains, such as healthcare, finance, and education, due to its privacy-preserving properties. However, implementing FL in dynamic wireless networks poses substantial communication challenges. Central to these challenges is the need for efficient communication strategies that can adapt to fluctuating network conditions and the growing number of participating devices, which can lead to unacceptable communication delays. In this article, we propose Stochastic Client Selection for Tree All-Reduce Federated Learning (CSTAR-FL), a novel approach that combines a probabilistic User Device (UD) sampling strategy with a treebased communication architecture to enhance communication efficiency in FL within densely populated wireless networks. By optimizing UD selection for effective model aggregation and employing an efficient data transmission structure, CSTAR-FL significantly reduces communication time and improves FL efficiency. Additionally, our approach ensures high global model accuracy under scenarios where data distribution is non-IID from UDs. Extensive simulations in dynamic wireless network scenarios demonstrate that CSTAR-FL outperforms existing state-of-the-art methods, reducing model convergence time by over 40% without losing the global model accuracy. This makes CSTAR-FL a robust solution for efficient and scalable FL deployments in high-density environments. |